Statistics-Based Trading Framework: A Comprehensive Guide for Systematic Trade Framing

In the ever-evolving landscape of financial markets, the ability to make informed and data-driven trading decisions is paramount. Statistics-based trading frameworks provide a structured and rigorous approach to analyze market data, identify trading opportunities, and manage risk. This comprehensive guide will delve into the intricacies of statistics-based trading frameworks, exploring their key components, methodologies, and applications in systematic trade framing.
Key Components of a Statistics-Based Trading Framework
1. Data Collection and Preprocessing: The foundation of any statistical analysis lies in the quality and relevance of the data. A robust statistics-based trading framework begins with the acquisition of reliable market data, which may include historical price movements, economic indicators, and market sentiment. Data preprocessing techniques, such as cleaning, filtering, and transformation, are essential to prepare the data for analysis.
5 out of 5
Language | : | English |
File size | : | 3143 KB |
Text-to-Speech | : | Enabled |
Screen Reader | : | Supported |
Enhanced typesetting | : | Enabled |
Word Wise | : | Enabled |
Print length | : | 17 pages |
Lending | : | Enabled |
2. Exploratory Data Analysis: Once the data is prepared, exploratory data analysis (EDA) is conducted to gain insights into its key characteristics. EDA techniques involve visualizing the data through charts and graphs, calculating summary statistics, and identifying potential patterns and outliers. This initial analysis helps traders understand the data's distribution, relationships, and potential for uncovering trading opportunities.
3. Hypothesis Development and Testing: Based on the results of EDA, traders can formulate hypotheses about the statistical relationships between market variables and trading outcomes. Statistical tests are then employed to evaluate the validity of these hypotheses. Hypothesis testing involves defining the null and alternative hypotheses, setting a significance level, and calculating the test statistic. If the test statistic falls within the critical region defined by the significance level, the null hypothesis is rejected, and the alternative hypothesis is deemed more likely to be true.
4. Model Building and Optimization: Validated hypotheses can be used to build statistical models that predict future market movements or identify trading opportunities. Model building involves specifying the input variables, selecting the appropriate statistical technique (e.g., regression, machine learning),and calibrating the model parameters. Optimization techniques are often used to fine-tune the model's performance and improve its accuracy.
5. Risk Management: Risk management is an integral part of statistics-based trading frameworks. Traders must assess the potential risks associated with trading strategies and implement measures to mitigate these risks. This includes setting stop-loss orders, diversifying portfolios, and calculating risk-adjusted performance metrics.
Methodologies for Systematic Trade Framing
Statistics-based trading frameworks provide a variety of methodologies for systematic trade framing, including:
1. Time Series Analysis: Time series analysis involves studying the historical behavior of market data over time. Techniques such as moving averages, exponential smoothing, and autoregressive integrated moving average (ARIMA) models are used to identify patterns, forecast future values, and generate trading signals.
2. Econometrics: Econometrics applies statistical methods to analyze economic data and build econometric models. These models can be used to estimate the relationships between economic variables and market movements, allowing traders to identify opportunities based on macroeconomic and financial factors.
3. Machine Learning: Machine learning algorithms can be harnessed to build complex statistical models that learn from historical data and make predictions about future market outcomes. Supervised learning techniques, such as linear/logistic regression, decision trees, and random forests, are commonly employed in statistics-based trading frameworks.
4. Statistical Arbitrage: Statistical arbitrage strategies exploit price discrepancies between different markets or instruments. By identifying and trading on these discrepancies, traders can generate profits while managing the risk associated with individual asset movements.
5. Basket Trading: Basket trading involves creating a portfolio of assets and trading them as a single unit. Statistical analysis can be used to select assets for the basket, optimize portfolio weights, and generate trading signals.
Applications of Statistics-Based Trading Frameworks
Statistics-based trading frameworks have numerous applications in systematic trade framing, including:
1. Trend Following: Identifying and following long-term price trends is a common application of statistics-based trading frameworks. Trend following strategies use statistical techniques to identify periods of sustained price momentum and generate trading signals accordingly.
2. Mean Reversion: Mean reversion strategies seek to profit from the tendency of prices to fluctuate around their long-term average. Statistical analysis is used to identify deviations from the mean and generate trading signals when the price is expected to revert to its average.
3. Swing Trading: Swing trading involves profiting from short-term price fluctuations within a larger trend. Statistics-based trading frameworks can be used to identify swing points and generate trading signals that capture these short-term price movements.
4. Volatility Trading: Volatility trading strategies exploit the fluctuations in market volatility. Statistical analysis is used to measure volatility, identify volatility regimes, and generate trading signals based on changes in volatility.
5. High-Frequency Trading: High-frequency trading involves executing a large number of trades in a short period of time. Statistics-based trading frameworks can be used to optimize order execution, manage risk, and generate trading signals for high-frequency trading strategies.
Benefits of Statistics-Based Trading Frameworks
1. Objectivity and Consistency: Statistics-based trading frameworks provide an objective and consistent approach to trading decisions, reducing the impact of biases and emotions.
2. Empirical Evidence: Statistical analysis is based on empirical evidence, ensuring that trading decisions are supported by verifiable data.
3. Optimization and Refinement: Statistical techniques allow traders to optimize and refine their strategies through backtesting and parameter tuning, improving their performance over time.
4. Automated Execution: Statistics-based trading frameworks can be automated, enabling traders to implement strategies efficiently and without manual intervention.
5. Risk Management and Control: Statistical analysis provides tools for risk assessment and management, helping traders to minimize losses and protect their capital.
Statistics-based trading frameworks offer a powerful and versatile approach to systematic trade framing. By leveraging statistical analysis, traders can gain valuable insights into market data, identify trading opportunities, manage risk, and make informed trading decisions. As the financial markets continue to evolve, statistics-based trading frameworks will undoubtedly play an increasingly important role in helping traders navigate the complexities of modern markets.
5 out of 5
Language | : | English |
File size | : | 3143 KB |
Text-to-Speech | : | Enabled |
Screen Reader | : | Supported |
Enhanced typesetting | : | Enabled |
Word Wise | : | Enabled |
Print length | : | 17 pages |
Lending | : | Enabled |
Do you want to contribute by writing guest posts on this blog?
Please contact us and send us a resume of previous articles that you have written.
Top Book
Novel
Fiction
Nonfiction
Literature
Paperback
Hardcover
E-book
Audiobook
Bestseller
Classic
Mystery
Thriller
Romance
Fantasy
Science Fiction
Biography
Memoir
Autobiography
Poetry
Drama
Historical Fiction
Self-help
Young Adult
Childrens Books
Graphic Novel
Anthology
Series
Encyclopedia
Reference
Guidebook
Textbook
Workbook
Journal
Diary
Manuscript
Folio
Pulp Fiction
Short Stories
Fairy Tales
Fables
Mythology
Philosophy
Religion
Spirituality
Essays
Critique
Commentary
Glossary
Bibliography
Index
Table of Contents
Preface
Introduction
Foreword
Afterword
Appendices
Annotations
Footnotes
Epilogue
Prologue
Maggie Nelson
Fujita
Laurie Pfalzer
Richard Kostelanetz
Dan Ames
Milen Slavov
E Chaton
James Barnes
Adam Alexander Haviaras
Barbara Sheklin Davis
Douglas E Richards
James Gleick
David Barnett
Adrian Rogers
R L Medina
Kevin Powell
Brenda Mohammed
Kevin Curry
Sharon Waters
Bobby Owsinski
Light bulbAdvertise smarter! Our strategic ad space ensures maximum exposure. Reserve your spot today!
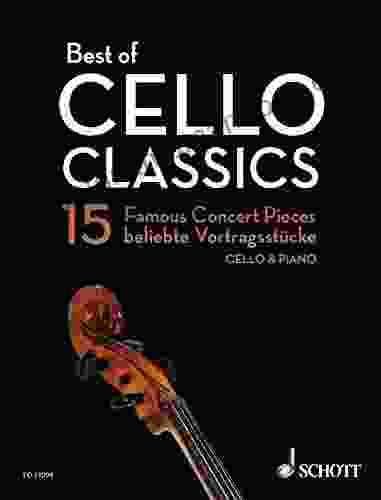

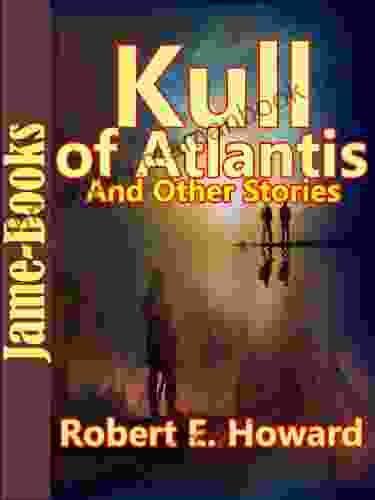

- Christian BarnesFollow ·6.3k
- Harvey HughesFollow ·17k
- Oscar WildeFollow ·2.5k
- Fyodor DostoevskyFollow ·6.7k
- Cody BlairFollow ·4.8k
- Aldous HuxleyFollow ·12k
- Edward ReedFollow ·9.7k
- Jacques BellFollow ·15.6k
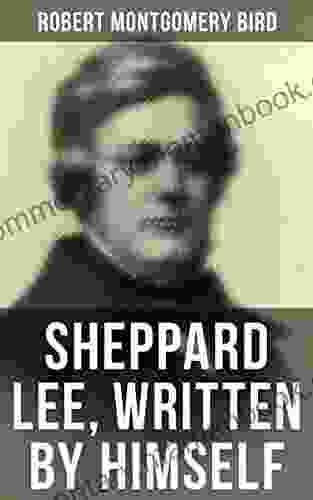

Sheppard Lee Written By Himself: A Journey of...
In the realm of...
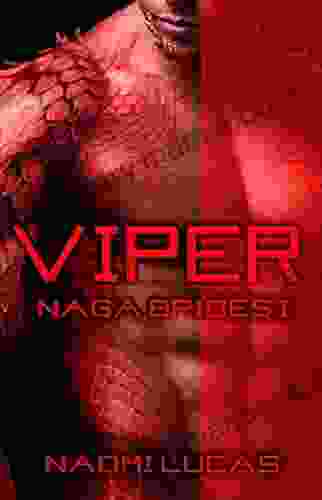

Viper Naga Brides: Unveiling the Enthralling Fantasy...
In the realm of...
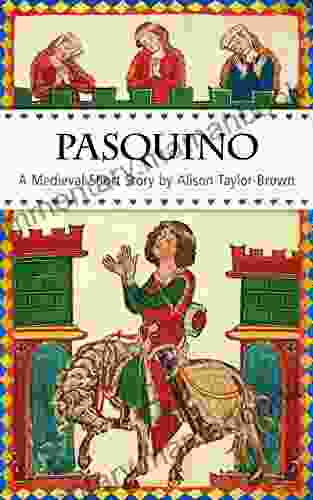

Once Upon a Hill in Tuscany: A Medieval Short Story
In the heart of medieval...
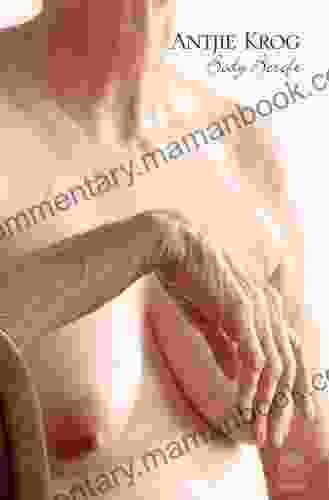

Body Bereft: Exploring Loss, Love, and Legacy in Antjie...
A Poetic Requiem for the Lost:...
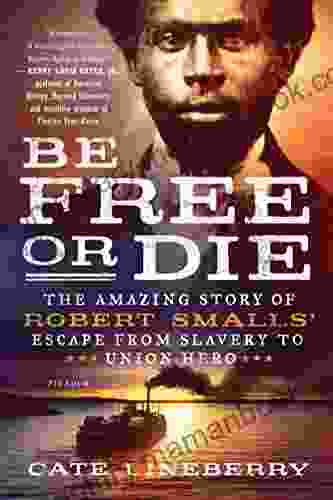

The Amazing Story Of Robert Smalls Escape From Slavery To...
The life of Robert Smalls is a testament to...
5 out of 5
Language | : | English |
File size | : | 3143 KB |
Text-to-Speech | : | Enabled |
Screen Reader | : | Supported |
Enhanced typesetting | : | Enabled |
Word Wise | : | Enabled |
Print length | : | 17 pages |
Lending | : | Enabled |